Abstract
Digital finance (DF) serves a pivotal role in facilitating the transition to green economies. While existing literature has explored the impact of DF on carbon emission reduction, the aspect of equity in carbon emissions has been largely neglected. Using a panel dataset of Chinese cities from 2011 to 2020, this study calculates the equitable industrial carbon emission (EICE) for 276 cities in China and quantitatively studies the effect of DF on EICE. The results indicate that DF significantly enhances EICE, with this effect being more pronounced in eastern regions and those with higher levels of internet development. Analysis of the mechanisms shows that DF achieves EICE through four pathways: industrial structure transformation, technological innovation acceleration, energy consumption reduction, and marketisation advancement. Additionally, this study confirms the positive moderating role of governmental environmental concern in strengthening the impact of DF on EICE, though the moderating effect of public environmental concern is not evident. This research provides new insights into evaluating the environmental benefits of the digital transformation of finance and the attainment of EICE.
Introduction
The urgency of climate change has intensified global efforts to mitigate pollution impacts, with CO2 emissions from anthropogenic activities being a key driver (Bamisile et al. 2020). As the world’s largest energy consumer and carbon emitter (Ritchie and Roser, 2024), China’s carbon emission reduction focuses on high-energy-consuming and high-polluting industrial sectors. From 1953 to 2006, the industrial sector, accounting for 40.1% of China’s GDP, was responsible for 67.9% of the nation’s energy consumption and 83.1% of its CO2 emissions (Chen, 2009). As the date for achieving the ‘dual carbon’ goalsFootnote 1 set by the Chinese government approaches, the traditional crude development model of high input, high consumption, and high pollution is increasingly unsustainable. Achieving a balance between economic growth and industrial carbon emission reduction has become an urgent challenge for China (Tu et al. 2022).
Research on industrial carbon emissions reduction is extensive (Zhang et al. 2022), but these studies neglect three critical issues related to carbon emissions. Firstly, the regional emission disparities have widened from 2005 to 2019 (Chen et al. 2023), coupled with a profit and loss deviation between carbon emissions and economic returns (Sun and Liu, 2016). The health burden of pollution is also unequally shared (Wang et al. 2022b). Secondly, to alleviate the pressures of rising energy demand and carbon emissions (Zhou et al. 2014), China has incrementally launched a series of pilot projects aimed at a market-oriented low-carbon economy, drawing attention to inter-regional carbon emission rights and responsibility sharing (Chen et al. 2023). A carbon quota allocation scheme that considers both equity and efficiency can mitigate regional abatement cost differences, thereby enhancing regional incentives for sustainable carbon reduction (Wang and Kong, 2022). Lastly, achieving the ‘dual carbon’ goals is challenged by the uneven resource distribution and economic development (Shi and Xu, 2023). Cities differ in their ability to reduce carbon emissions, and failures in less developed regions may hinder China’s overall progress (Liu and Xu, 2023). Especially with the rise of ‘inclusive growth’, the model of growth with equal economic and social opportunities (equitable growth) has gained wider acceptance among social groups. From the perspective of aligning authority with responsibility, identifying non-governmental entities that can simultaneously enhance the efficiency and equity of carbon emissions will contribute to inclusive growth, enabling China to achieve ‘dual carbon’ goals on schedule while also providing a foundation for governments worldwide to develop effective environmental policies.
The transition towards a low-carbon society depends on both guidance from governmental regulations and support from finance (Razzaq and Yang, 2023). However, the traditional financial system faces many limitations in regulatory burdens and credit coverage (Buchak et al. 2018). As a vital component of transitional finance, digital finance (DF) supports the low-carbon transformation by establishing efficient and sustainable financial models (Li et al. 2023). Over the past decade, China has become one of the leading countries in DF (Wu and Huang, 2022). The ‘14th Five-Year Digital Economy Development Plan’ of China underscores the urgency to ‘accelerate the digital transformation of financial sectors’ (Zhang, 2023).
Given the inevitable trend towards digital transformation in finance, does DF improve or exacerbate equitable industrial carbon emissions (EICE)? What forms of heterogeneity do these impacts exhibit? What are the specific mechanisms through which DF affects EICE? What impact do different social participants have on the relationship? Current research addresses the relationship between DF and carbon emissions, as well as energy justice. Studies that empirically test the relationship between DF and EICE are to be complemented.
This study aims to establish three marginal contributions. Firstly, it provides a new perspective on the interplay between digital transformation in finance and low-carbon transformation in the industrial sector, broadening the scope of literature on the pollution haven and presenting new evidence of the environmental benefits yielded by DF. Secondly, diverging from existing research that focuses solely on technological innovation or industrial transformation, this study employs energy consumption, which directly affects carbon emissions, and the marketisation level, which influences factor mobility, as mediating variables. These insights provide theoretical underpinnings to strengthen the positive impacts of DF on carbon reduction. Thirdly, based on the attention theory, this study incorporates social participants into the analytical framework by introducing governmental environmental concern and public environmental concern, which makes it possible to systematically examine the effects of different social participants’ environmental concerns on the relationship between DF and EICE.
The remainder of this paper is structured as follows: Section 2 elucidates the theoretical analysis and hypotheses. Section 3 introduces the methods and data. Section 4 presents the baseline results and robustness tests. Section 5 details the heterogeneity analysis, mediating effects, and moderating effects. Finally, the conclusions and policy recommendations are provided.
Theoretical analysis and hypothesis development
With its inherent digital and financial characteristics, DF enhances the unhindered flow and efficient allocation of capital, information, and digital resources, addressing market inefficiencies and financial disconnects due to information asymmetry (Zhang, 2023). Hence, DF constitutes one of the primary drivers of economic growth (Sethi and Acharya, 2018). With the rapid development of the digital economy, the integration of DF with the real economy has deepened (Liu et al. 2021). Scholars have found that on the production side, DF helps increase gross fixed capital formation (Qin et al. 2021). DF can accurately provide financial services to enterprises using green technologies (Ren et al. 2023) and promote the digitalization of enterprises (Razzaq and Yang, 2023). Consequently, the enhancement of regional intelligent management capabilities is observed, leading to improvements in EICE. From the consumer perspective, DF can improve household payment convenience and generate wage premiums (Wang et al. 2022a), which enhances cross-regional consumption of products and therefore improves EICE.
Hypothesis 1: There is a positive relationship between DF and EICE.
Following the logic of industrial structure changes induced by the development of DF—industrial structural transformation, the low-carbon transformation behaviours of enterprises that emit industrial carbon—technological innovation, the usage of energy that is directly related to carbon emissions—energy consumption, and the external factors affecting the resources mobility—marketisation level, the mechanism of DF on EICE is proposed.
Divergent industrial structures contribute to varying levels of carbon emissions and pollution. Typically, carbon emission intensity is generally believed to be negatively correlated with the proportion of the tertiary sector in GDP (Zhang et al. 2014), while an industrial development pattern dominated by heavy industry is not conducive to improving EICE (Liu and Xu, 2023). Past research has underscored the significant role that the traditional finance sector plays in driving industrial structural upgrades (Sasidharan et al. 2015). Leveraging its cost-cutting benefits and broad applicability, DF stands poised to address deficiencies inherent in the traditional financial sector, thereby amplifying its role in industrial optimization. Additionally, the advantages of DF in terms of enabling optimal risk management can effectively direct societal capital towards high-tech industries, reshaping the direction of industrial growth (Nie et al. 2021) and consequently promoting the transformation of industrial structure.
Hypothesis 2: Industrial upgrading serves as a significant mediating variable between DF and EICE.
Technological innovation represents a crucial approach for realizing the green transition (Ouyang et al. 2020). DF markedly encourages innovation within enterprises. Firstly, DF is characterized by its technology-driven nature. Therefore, the advanced technologies applied by DF, such as big data and artificial intelligence, demand higher workforce quality and digitalization levels within enterprises. Additionally, DF can alleviate the financing constraints of enterprises (Liu and Xu, 2023), increasing the possibility of investment in technological innovation. Lastly, compared to traditional finance, DF provides a lower-cost platform and promotes technological exchange among enterprises. DF facilitates technology spillover effects through industrial chain embedding, knowledge transfer, and other methods, offering a comprehensive advantage in fostering innovation and technological advancement (Zhu et al. 2024).
Hypothesis 3: Technological innovation serves as a significant mediating variable between DF and EICE.
Irrational energy consumption patterns lead to an increased rate of carbon emissions that surpasses the rate of carbon absorption, which results in a reduction in EICE. In the context of energy substitution, DF plays a role in steering the real economy towards low-carbon patterns. As a result, the coverage cost of clean energy tools is effectively reduced, and the substitution of new energy for high-carbon energy is accelerated (Razzaq and Yang, 2023). These processes contribute to the overall energy transition. In terms of information efficiency, enterprises have built a series of online trading platforms based on DF, breaking through the limitations of time and space (Zhao et al. 2021), which reduces the energy consumption of information flow processes.
Hypothesis 4: Energy consumption serves as a significant mediating variable between DF and EICE.
Digitalization benefits from overcoming temporal and spatial limitations, which boosts the efficiency of financial services (Lee et al. 2022). It also breaks the monopoly of large banks to a certain extent, increasing the level of competition in the financial sector. This competitive landscape enhances resource allocation efficiency in urban areas and elevates the marketisation level. DF plays a pivotal role in directing funds towards green credit and green bonds, thereby weakening the market segmentation caused by geographical distance. The free flow of production factors reduces the financing costs of low-polluting enterprises and curtails financing activities for high-pollution ones. Consequently, DF supports the advancement of EICE, promoting a more sustainable and environmentally friendly economic landscape (Han et al. 2024).
Hypothesis 5: Marketisation level serves as a significant mediating variable between DF and EICE.
When examining the social participation actors involved in carbon emission reduction, our analysis includes perspectives from both the government and the public, dividing social environmental consciousness into two distinct dimensions (Mao and Wang, 2023): governmental environmental concern and public environmental concern. This bifurcation offers a nuanced understanding of how different segments of society contribute to and perceive efforts towards mitigating carbon emissions, highlighting the critical roles of both governmental policies and public concern in advancing environmental sustainability.
Governmental environmental concern further strengthens the improvement of DF on EICE. Drawing from attention theory, when policymakers concentrate on low-carbon transition, enterprises are encouraged to align their profit-maximization efforts with green development objectives (Tang et al. 2024). Local governments possess authoritative enforcement and policy implementation capabilities. By employing the ‘visible hand’, the government can allocate financial resources and mitigate distortions in factor allocation caused by environmental externalities. Evidence suggests that stringent environmental regulations catalyse increased corporate investments in green initiatives, demonstrating a reciprocal reinforcement between regulatory rigour and enterprises’ commitment to sustainability (Zhang et al. 2016). For enterprises, the government’s emphasis on green and low-carbon development reduces the uncertainty associated with innovation activities. Enterprises are thus incentivized to invest in research and development (R&D) through green investments and leveraging government subsidies, among other strategies. This approach not only lessens the financial burden associated with corporate emissions but also advances the realization of a green and low-carbon environmental ethos.
Hypothesis 6: The improvement in EICE through DF is more pronounced when governmental environmental concern is high.
Besides governmental environmental concerns, public environmental concern, acting as an informal supervision, also affects the impact of DF on EICE. The online payment platforms built by DF, which the public uses daily, can provide additional capital for DF, alleviating the financing constraints that institutions encounter in their low-carbon transformation. Serving as an augmentation to governmental efforts, the increase in public demand for low-carbon solutions and environmental consciousness will drive citizens to supervise enterprises’ green production through monitoring, reporting, and public opinion (Deng and Zhang, 2022), thereby encouraging enterprises to reduce carbon emissions. Lastly, the public’s preference for consuming green products signals financial markets to favour green investments, potentially increasing corporate green investments (Liao and Shi, 2018) and consequently enhancing the EICE.
Hypothesis 7: The improvement in EICE through DF is more pronounced when public environmental concern is high.
Based on the theoretical analysis, the conceptual framework is shown in Fig. 1.
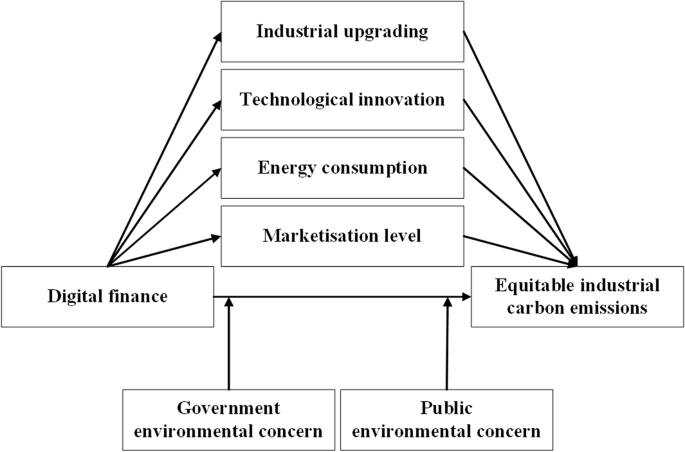
The conceptual model proposes that digital finance directly promotes equitable industrial carbon emissions, mediated through industrial structural transformation, technological innovation acceleration, energy consumption reduction, and marketization advancement. Government environmental concern and public environmental concern moderate the strength of digital finance’s impact on the equitable industrial carbon emissions. Control variables are omitted for schematic clarity but included in empirical analyses.
Research design
Variable construction
Equitable industrial carbon emission (EICE)
According to carbon emission equity theory from Rose et al. (1998), carbon emission allocation method from Song et al. (2017), and variable settings by Shi et al. (2023), this study defines the proxy for EICE as a corrected indicator of industrial carbon emission intensity that accounts for inter-city equity, referred to as equitable industrial carbon emission intensity (EICEI).
Where ({{IGDP}}_{i,t}) represents industrial GDP of city (i) in year (t), ({{ICE}}_{i,t}) denotes industrial carbon emissions, and ({{POP}}_{i,t}) is population. ({sigma }_{i,t}) signifies the equity distribution index, (max {sigma }_{t}) and (min {sigma }_{t}) represent the highest and lowest values of the fairness distribution index in period (t). It is important to note that ({EICEI}) is a negative indicator. A larger value of EICEI suggests that the city bears a higher responsibility for reducing industrial carbon emissions after adjusting for the equity index and thus implies a lower EICE.
Digital finance
The DF indicator in this paper broadly refers to traditional financial institutions and internet companies utilizing digital technology for financing, payments, investments, and other new financial enterprise models (Huang and Huang, 2018). Considering the measurement methods and data limitations of city-level samples (Mao and Wang, 2023), the data source for DF in this paper is the Peking University Digital Financial Inclusion Index of China (PKU_DFIIC), which is co-compiled by the Digital Finance Research Center of Peking University and Ant Financial Enterprise.
Mediating variable
The study identifies four mediating variables: industrial structure transformation (({Ind})), technological advancement (({Tec}h)), energy consumption (({Enc})), and marketisation level (({Mar})). The industrial structure (({Ind})) is defined as the ratio of gross output of the tertiary industry to gross output of the secondary industry. The higher the value of ({Ind}), the larger the proportion of the tertiary sector within the city. Technological advancement is commonly measured by the number of patents in a city, which in this paper is expressed as the logarithm of the number of design patents filed in the year plus one. ({Enc}) is measured by the total energy consumption of the city. The level of marketisation is defined by the marketisation index provided in the Marketisation Index of China’s Provinces (Wang et al. 2021), which comprehensively reflects the level of marketisation in each city in China from five dimensions: the relationship between the government and the market, the development of the non-state-owned economy, the degree of development of the product market, the degree of development of the factor market, the development of market intermediary organizations, and the legal system environment.
Moderating variable
Referring to the study of Wang et al. (2024), this paper employs the word frequency statistics method to analyse city government work reports. The proportion of four keywords—environmental pollution, synergistic development and environmental co-management, green living, and environmental institution building—relative to the total word count of the reports is used as the indicator of governmental environmental concern (GEC). Inspired by Lu and Wu (2023), this paper captures the search frequency of the keyword ‘industrial pollution’ across various cities from 2011 to 2020 using the Baidu Index search engine. The normalised data are used as a proxy variable for public environmental concern (PEC).
Control variable
As outlined previously, the factors affecting EICE are multidimensional. To minimize the endogeneity problems caused by unobservable factors, this paper introduces economic development (Lngdp), financial development (Fin), government finance (Gov), education investment (Lnedu), and technological investment (Lnsci) as control variables. The economic development is measured by the logarithm of the Gross Domestic Product (GDP), while the financial development is measured by the ratio of the total year-end RMB deposit balances to the total year-end RMB loan balances at financial institutions. Government finance is measured by the ratio of local general public budget revenues to local general public budget expenditures. Education investments and technological investments are represented by the logarithms of local government expenditures on education and technology.
Data sources
This study primarily employs panel data from 276 cities in China, covering the period from 2011 to 2020. The data are mainly obtained from the China Urban Statistical Yearbook, statistical yearbooks of provinces or cities, the Peking University Digital Inclusive Finance Index (2011–2020), and the China Provincial Marketisation Index Report. The GEC and PEC are manually collected from city government reports and Baidu Index. Missing data are filled in with the interpolation method. Descriptive statistics for variables, including definitions, means, and standard deviations, are displayed in Table 1.
Model setting
Baseline regression based on the two-way fixed effects panel model
As a preliminary exploration of the relationship between DF and EICE, this paper constructs a panel regression model incorporating both time-fixed effect and individual fixed effect. The baseline model is set up as shown in Eq. (3).
({{EICEI}}_{i,t}) denotes the equitable industrial carbon emission intensity for city (i) in year (t), which is the main dependent variable in this paper. ({{DF}}_{i,t}) represents the level of digital finance. ({{Control}}_{i,t}) encompasses a set of control variables. ({mu }_{i}) accounts for the city fixed effect, ({lambda }_{t}) captures the year fixed effect, and ({varepsilon }_{i,t}) represents the random disturbance term. Since EICEI is a negative indicator compared to EICE, a significantly negative coefficient ({alpha }_{1}) of DF indicates that an increase in DF effectively reduces EICEI. In other words, DF has a positive impact on EICE, thereby supporting Hypothesis 1. Additionally, the baseline model is also used in two heterogeneity analyses and three robustness tests: substituting the measurement of the explanatory variable DF, winsorizing the data of DF and EICE, and excluding samples from municipalities directly under central government control.
Instrumental variable method with two-stage least squares (IV-2sls) and generalised method of moments (GMM) models
This study focuses on the relationship between DF and EICE, which presents potential endogeneity in Eq. (3). First, the numerous factors affecting EICE imply the control variables selected for this study are limited, which could lead to omitted variable bias. Second, reverse causality may occur if cities with better EICE facilitate the development of DF. To address these concerns, this study employs IV-2sls and GMM models to mitigate the bias in the baseline regression (Song et al. 2021; Fan and Feng, 2022). The models are specified in Eqs. (4)–(6).
Equations (4) and (5) illustrate the estimation model of the IV-2sls method. The core idea is to eliminate the correlation between DF and the error term by introducing an instrumental variable (IV) that is correlated with DF but uncorrelated with the error term. The predicted (widehat{{DF}}) is substituted as a new explanatory variable into Eq. (5) for the second stage of regression to obtain an unbiased estimator.
The GMM model in Eq. (6) is estimated by constructing a set of moment conditions using multiple instrumental variables. This estimation method mitigates the potential serial correlation and endogeneity issues related to industrial carbon emission inequity among cities and further improves the accuracy and robustness of the estimation (Song et al. 2021).
Mediation model
The mediation model offers deeper insights into the mechanisms through which DF affects EICE, helping to clarify the relationship. Drawing on Wang et al. (2024), this study constructs the following mediation model.
({{ME}}_{i,t}) represents the mediating variable, including industrial structure transformation, technological advancement, energy consumption, and the level of marketisation. All other specifications remain consistent with the baseline regression. If ({beta }_{1}) is significant, it indicates that DF effectively influences the mediating variable, establishing the first stage of the mediating effect. Further, if ({gamma }_{2}) is also significant, it indicates that the mediating variable influences EICEI, confirming the second stage of the mediating effect. Therefore, if both ({beta }_{1}) and ({gamma }_{2}) are significant, it proves that this mediating mechanism is valid, and the mediating effect in Hypothesis 2-Hypothesis 4 is supported.
Moderation model
Drawing on Li et al. (2021), the following moderation model is constructed to examine whether environmental concerns from various social parties moderate the relationship between DF and EICE. The corresponding model is shown in Eq. (9).
Where ({{MO}}_{i,t}) represents the moderating variable, including the governmental environmental concern and public environmental concern. Other variables are specified in the same way as in Eq. (3). If ({varphi }_{3}) in Eq. (9) is significant, it suggests that environmental concern significantly moderates the relationship between DF and EICE, thus supporting H6 and H7.
Empirical analysis
Baseline analysis
Based on the baseline model in Eq. (3), the relationship between DF and EICE is reported in Table 2. In the absence of any control variables or fixed effects, the coefficient of DF on EICEI is −0.034. The Hausman test provides empirical support for the choice of a fixed-effect model. With the inclusion of year fixed effect and city fixed effect, the sign of the coefficient of DF on EICEI remains negative. Subsequently, control variables and fixed effects are added to the model, and the sign of the estimated coefficient of DF remains stable and significantly negative ((beta =-0.221,{p} ,<, 0.01)). For every 1 unit increase in DF, the EICEI decreases by 0.221 units, which means that EICE increases by 0.221 units, and H1 is supported. Combining the results of stepwise regression in Table 2, the coefficient of DF on EICE remains significant at the 5% level. The value of R2 increases gradually, indicating that the above estimation results have a high degree of robustness and Hypothesis 1 is supported.
Robustness test
In this section, this paper conducts a series of robustness tests to verify the robustness of the baseline regression results.
IV-2sls test
The spherical great-circle distance of each city from Hangzhou (({Dis})) is considered as the IV. The distance variable naturally satisfies the condition of being uncorrelated with the error term (Li et al. 2023). Furthermore, Alibaba, located in Hangzhou, is the leader in DF in China, and the diffusion of DF has been argued to be affected by geographical distance (Guo et al. 2017). Therefore, the IV satisfies the relevance condition with DF. Given that the actual regression uses panel data, where the distance between cities remains constant over time, this paper defines the IV as the product of the spherical straight-line distance between the city and Hangzhou and the lagged term of DF (({Dis}times L.{DF})). The regression is performed according to the model set in Eqs. (4) and (5), and results are shown in column (1) of Table 3.
According to Table 3, the p-value of the Kleibergen-Paap rk LM statistic is 0, indicating that the null hypothesis of insufficient instrumental variable identification can be rejected at the 1% level. Both the Cragg-Donald Wald F statistic and the Kleibergen-Paap Wald rk F statistic are well above the critical value of 16.38 for a 10% bias, showing that the weak instrumental variable issue does not exist, and there is a strong correlation between IV and the endogenous explanatory variable. The estimated regression coefficient is −0.157, which is statistically meaningful at the 5% level, confirming that the baseline result remains valid after accounting for potential endogeneity.
GMM model
The results of GMM model regression based on the model of Eq. (6) are shown in column (2) of Table 3. The results show that AR (1) is less than 0.1 and AR (2) is greater than 0.1, supporting the null hypothesis of without second-order autocorrelation. The Hansen test result is greater than 0.1, validating the outcomes of GMM model. The significance of DF at the 10% level indicates that the improvement effect of DF on EICE persists after adjusting for serial correlation in EICE. The conclusion from baseline regression is corroborated.
Replace independent variable
This paper uses the Peking University Digital Inclusive Finance Index (2011–2020) as the source for DF. The single source of data may impact our analysis results. To avoid potential biases, the independent variable is replaced by digital economy (DE) (Bai and Yu, 2021). DE is derived through principal component analysis from four dimensions: digital users, digital enterprises, digital platforms, and digital outputs. Column (3) of Table 3 reports the regression results after replacing the core independent variable DF in Eq. (3) with DE. The results show that the coefficient of DE on EICEI is negative, reaffirming the validity of the previous conclusion.
Winsorizing DF and EICEI
To mitigate the influence of potential outliers, this paper performed 1% winsorization on both the core independent and dependent variables. The winsorized data are subjected to regression analysis based on Eq. (3). The outcomes in column (4) of Table 3 show that for every 1 unit increase in DF, EICE enhances by 0.19 units. These results align with the baseline findings, suggesting that the conclusions are robust from the standpoint of data reliability.
Excluding municipalities
There are differences between municipalities directly under the central government and other cities in terms of economic scale, population distribution, and geographical scope. This study further regresses the panel data of 272 cities using Eq. (3), excluding the four municipalities (Beijing, Tianjin, Shanghai, and Chongqing). The regression results in column (5) of Table 3 show that after removing these four municipalities, the estimator and significance of DF on EICE remain largely unchanged. The effect of DF on EICE is robust.
Further analysis
Heterogeneity analysis
EICE reflects regional disparities in carbon emissions, influenced by differences in resource endowment and economic development levels, which may affect the role of DF. In addition, the main explanatory variable in this paper is DF, whose role depends on the development of digital technology and the internet. The differences in internet penetration across regions may cause DF to show different effects in promoting EICE. Applying the baseline two-way fixed effects model to samples divided by geographic location and internet development provides insights into the heterogeneous relationship between DF and EICE.
Geographic heterogeneity analysis
Columns (1) and (2) of Table 4 show regression results based on Eq. (3), where the sample of 276 cities is divided into eastern and midwestern cities. It is evident that DF can improve EICE between cities, both in eastern and midwestern cities. This effect is −0.354 in eastern cities, with the impact coefficient negative at the 5% level. In midwestern cities, the estimator is merely −0.036 and fails the significance test. The relationship between DF and EICE exhibits marked regional heterogeneity. One possible reason is that, compared to central and western cities, the eastern cities have better economic foundations, more high-quality labour, accessible transportation, and predominantly knowledge-intensive industrial layouts. Enterprises in the eastern cities have smoother access to financing, encounter less emission reduction pressure, receive more substantial fiscal support from local governments, and are superior to less developed areas in both development opportunities and institutional environments (Wu et al. 2020). Hence, DF has a more pronounced effect on enhancing EICE in eastern cities compared to the central and western cities.
Internet development heterogeneity analysis
The number of urban internet access users serves as a proxy for internet development. The second heterogeneity analysis divides the sample by the mean number of internet broadband subscribers, separating cities with higher internet penetration rates and lower internet penetration rates. The baseline model (Eq. 3) is applied to each group separately. The regression results in columns (3) and (4) of Table 4 indicate that in cities with higher internet penetration, EICEI is significantly reduced by 0.448 units for every 1 unit increase in DF ((p ,<, 0.05)), which also implies a 0.448 unit increase in EICE. In contrast, in cities with lower internet penetration, the positive contribution of DF on EICE is only 0.179. The reason might be that high internet penetration necessitates robust digital infrastructure, laying the foundational support for DF’s emergence. Furthermore, internet usage enhances regional familiarity with digital technology knowledge, creating a market foundation for the adoption of DF. Therefore, compared to areas with a low level of internet development, the improvement effect of DF on EICE is more pronounced in areas with a higher level of internet development.
Mediating effect
The mediating variables represent channels through which DF affects EICE. In the following section, in order to test Hypothesis 2-Hypothesis 5, the mediation model constructed by Eqs. (7) and (8) are used to test the validity of the four mediating variables.
Industrial structural transformation
The mediation tests show that industrial structural transformation plays a significant mediating role between DF and EICE. Specifically, in column (1) of Table 5, the impact of DF on Ind is positive at the 5% level, which implies that DF can effectively promote an increase in the proportion of tertiary industries in the city’s industrial structure, and the first stage of the mediating effect is valid. In the second stage of the mediation model, the impact of industrial structural transformation is significant at the 1% level, and DF is still significantly negative ((beta =-0.144,{p}, <, 0.05)), which implies that DF promotes EICE through industrial structural transformation. These results support Hypothesis 2 and also further validate the positive role of DF in promoting the transformation of the industrial sector.
Technological innovation
The regression results presented in columns (3) and (4) of Table 5 utilise technological innovation as the mediating variable. Column (4) shows that a 1 unit increase in technological innovation raises EICE by 0.024 units, and this finding is significant at the 10% level. Compared with the DF coefficient of −0.221 in the baseline regression, the explanatory power of DF on EICE slightly decreases after adding technological innovation, a phenomenon that suggests that technological progress plays a partially mediating role between DF and EICE. This further validates Hypothesis 3, suggesting that promoting technological innovation not only enhances the city’s innovation capacity but also plays a key role in promoting EICE.
Energy consumption
The analyses of mediating roles on energy consumption are presented in Table 5. Column (5) shows that DF significantly reduces regional energy consumption ((beta =-2.880,{p} ,<, 0.01)). Column (6) reveals that for every 1 unit reduction in energy consumption, the EICE in cities can be effectively improved by 0.013 units. The regression coefficient of DF is notably negative ((beta =-0.182,{p} ,<, 0.05)), indicating that energy consumption has a partial mediating effect. In summary, DF reduces EICEI through optimising energy consumption, supporting Hypothesis 4.
Marketisation level
The results of the regression with marketisation as a mediating variable show that it plays a positive mediating role between DF and EICE. The coefficient of DF on the marketisation index in column (7) of Table 5 is 0.670, significant at the 1% level, indicating that DF enhances city marketisation. The effect of marketisation level on EICEI in column (8) is significant ((beta =-0.064,{p} ,<, 0.01)), which suggests that the promotion of marketisation reforms is an effective means of achieving EICE. Combined with the coefficient of DF on EICE of 0.178, it indicates that DF can promote EICE in cities by enhancing the city’s marketisation level, and Hypothesis 5 is supported.
Moderating effect
Governmental environmental concern
The regression results for the moderating effect of governmental environmental concern, as reported in column (2) of Table 6, show that the regression coefficient for the interaction term ({DF}times {GEC}) is −0.035. This indicates that governmental environmental concern can effectively strengthen the impact of DF on EICE, and Hypothesis 6 is supported. Specifically, higher governmental concern for environmental protection enhances the positive effect of DF on EICE. This suggests that policy support and regulatory efforts from the government play an important role in enhancing DF for EICE.
Public environmental concern
The regression results with public environmental concern as a moderating variable are shown in column (3) of Table 6. The coefficient of the interaction term between DF and public environmental concern, ({DF}times {PEC}), is negative, indicating that the impact of DF on EICE is stronger when public environmental concern is high. Unfortunately, the moderating impact is limited, as the interaction term is statistically insignificant ((beta =-0.020,{p}=,0.17)). This limited moderating effect may arise due to the transformation from environmental willingness to protection behaviour, which is affected by various factors (Liu and Mu, 2016). While the public expresses concern for environmental issues, there can be a gap between awareness and actual actions, which limits the role of public environmental concern in promoting the relationship. Additionally, public environmental concern is often carried out through informal channels, and there are limited environmental regulation tools for the public (Lu and Wu, 2023). Consequently, the moderating effect of public environmental concern is not significant, and Hypothesis 7 is not supported.
Conclusion
While reducing the total industrial carbon emissions is the most direct approach to achieving dual carbon goals, ensuring equitable industrial carbon emissions across regions is also essential from a welfare perspective. This paper uses panel data for 276 Chinese cities from 2011 to 2020 to empirically explore the relationship between DF and EICE. The findings indicate that DF positively enhances EICE, especially in eastern regions and cities with higher internet penetration. Industrial transformation, technological innovation, reduced energy consumption, and improved marketisation are effective pathways. Moreover, governmental and public environmental concerns amplify the positive impact of DF on EICE. However, the moderating effect of public environmental concern is statistically insignificant, indicating that public participation in environmental protection requires further improvement.
Based on these findings, the hypotheses, empirical results, and policy implications of this paper are summarised in Table 7 to provide a clearer overview of the study.
Limitation and future research
This paper discusses the impacts, mediating variables, and moderating variables of DF on EICE. Given the non-significant moderating impact of public environmental concern, this raises questions about how to more effectively translate public environmental awareness into action and expand the range of tools for public environmental engagement. Future research could investigate strategies to enhance public environmental participation. Additionally, data constraints limit this study, preventing analysis of EICE at the enterprise level. With advancements in big data, future studies could conduct a more detailed examination of EICE from a finer-grained perspective.
Data availability
The data that support the findings of this study are available on request from the corresponding author upon reasonable request.
Notes
-
In the General Debate of the 75th session of the United Nations General Assembly, Chinese President Xi Jinping proposed a ‘two-step’ goal of achieving carbon peaking by 2030 and carbon neutrality by 2060.
References
-
Bai PW, Yu L (2021) Digital economy development and firms’ markup: theoretical mechanisms and empirical facts. China Ind Econ 11:59–77. https://doi.org/10.3969/j.issn.1006-480X.2021.11.004
Google Scholar
-
Bamisile O, Obiora S, Huang Q et al. (2020) Towards a sustainable and cleaner environment in China: dynamic analysis of vehicle-to-grid, batteries and hydro storage for optimal RE integration. Sustain Energy Technol Assess 42:100872. https://doi.org/10.1016/j.seta.2020.100872
Google Scholar
-
Buchak G, Matvos G, Piskorski T, Seru A (2018) Fintech, regulatory arbitrage, and the rise of shadow banks. J Financ Econ 130(3):453–483. https://doi.org/10.1016/j.jfineco.2018.03.011
Google Scholar
-
Chen C, Gao ZH, Wang ZB (2023) Analysis on influencing factors of industrial carbon emission and carbon transfer pattern at provincial level. Acta Ecol Sin 43(14):5816–5828. https://doi.org/10.5846/stxb202205291509
Google Scholar
-
Chen SY (2009) Energy consumption, CO2 emission and sustainable development in Chinese industry. Econ Res J 44(04):41–55
Google Scholar
-
Deng RR, Zhang AX (2022) Research on the impact of urban digital economy development on environmental pollution and its mechanism. South China J Econ 2:18–37. https://doi.org/10.19592/j.cnki.scje.390724
Google Scholar
-
Fan QQ, Feng SX (2022) Mechanism and effects of digital finance on carbon emissions. China Popul Resour Environ 32(11):70–82. https://doi.org/10.12062/cpre.20220920
Google Scholar
-
Guo F, Kong T, Wang JY (2017) The spatial agglomeration effect of internet finance——evidence from the financial development index. Stud Int Financ 8:75–85. https://doi.org/10.16475/j.cnki.1006-1029.2017.08.008
Google Scholar
-
Han X, An R, Huang X (2024) How digital finance promotes high-quality economic development: theoretical mechanism and empirical test. Financ Regul Res 1:79–95. https://doi.org/10.3969/j.issn.2095-3291.2024.01.005
Google Scholar
-
Huang YP, Huang Z (2018) The development of digital finance in China: present and future. China Econ Q 17(4):1489–1502. https://doi.org/10.13821/j.cnki.ceq.2018.03.09
Google Scholar
-
Lee CC, Wang FH, Lou RC (2022) Digital financial inclusion and carbon neutrality: evidence from non-linear analysis. Resour Policy 79:102974. https://doi.org/10.1016/j.resourpol.2022.102974
Google Scholar
-
Li GX, Wu HY, Jiang JS, Zong QQ (2023) Digital finance and the low-carbon energy transition (LCET) from the perspective of capital-biased technical progress. Energy Econ 120:106623. https://doi.org/10.1016/j.eneco.2023.106623
Google Scholar
-
Li XL, Li CH, Wang Z et al. (2021) The effect of corporate philanthropy on corporate performance of Chinese family firms: the moderating role of religious atmosphere. Emerg Mark Rev 49:100757. https://doi.org/10.1016/j.ememar.2020.100757
Google Scholar
-
Liao XC, Shi XP (2018) Public appeal, environmental regulation and green investment: evidence from China. Energy Policy 119:554–562. https://doi.org/10.1016/j.enpol.2018.05.020
Google Scholar
-
Liu SC, Yan JC, Zhang SX, Lin HC (2021) Can corporate digital transformation promote input-output efficiency? J Manag World 37(5):170-190+13. https://doi.org/10.3969/j.issn.1002-5502.2021.05.014
Google Scholar
-
Liu XS, Mu R (2016) Public environmental concern in China: determinants and variations. Glob Environ Change 37:116–127. https://doi.org/10.1016/j.gloenvcha.2016.01.008
Google Scholar
-
Liu ZH, Xu JW (2023) Equity and influence factors of China’s provincial carbon emissions under the “Dual Carbon” goal. Sci Geogr Sin 43(1):92–100. https://doi.org/10.13249/j.cnki.sgs.2023.01.010
Google Scholar
-
Lu HY, Wu ZF (2023) Relationship between energy-consuming right trading system and low-carbon transformation of energy consumption structure. Resour Sci 45(6):1181–1195. https://doi.org/10.18402/resci.2023.06.0
Google Scholar
-
Mao XM, Wang RZ (2023) Digital finance and green development——empirical evidence from 286 cities in China. Financ Forum 28(9):69–80. https://doi.org/10.16529/j.cnki.11-4613/f.2023.09.006
Google Scholar
-
Nie XH, Jiang P, Zheng XJ, Wu Q (2021) Research on digital finance and regional technology innovation. J Financ Res 3:132–150
Google Scholar
-
Ouyang XL, Li Q, Du KR (2020) How does environmental regulation promote technological innovations in the industrial sector? Evidence from Chinese provincial panel data. Energy Policy 139:111310. https://doi.org/10.1016/j.enpol.2020.111310
Google Scholar
-
Qin LG, Raheem S, Murshed M et al. (2021) Does financial inclusion limit carbon dioxide emissions? Analyzing the role of globalization and renewable electricity output. Sustain Dev 29(6):1138–1154. https://doi.org/10.1002/sd.2208
Google Scholar
-
Razzaq A, Yang XD (2023) Digital finance and green growth in China: appraising inclusive digital finance using web crawler technology and big data. Technol Forecast Soc Change 188:122262. https://doi.org/10.1016/j.techfore.2022.122262
Google Scholar
-
Ren XH, Zeng GD, Zhao Y (2023) Digital finance and corporate ESG performance: empirical evidence from listed companies in China. Pac-Basin Financ J 79:102019. https://doi.org/10.1016/j.pacfin.2023.102019
Google Scholar
-
Ritchie H, Roser M (2024) CO2 emissions. Our World in Data
-
Rose A, Stevens B, Edmonds J, Wise M (1998) International equity and differentiation in global warming policy. Environ Resour Econ 12(1):25–51. https://doi.org/10.1023/A:1008262407777
Google Scholar
-
Sasidharan S, Jijo Lukose PJ, Komera S (2015) Financing constraints and investments in R&D: evidence from Indian manufacturing firms. Q Rev Econ Financ 55:28–39. https://doi.org/10.1016/j.qref.2014.07.002
Google Scholar
-
Sethi D, Acharya D (2018) Financial inclusion and economic growth linkage: some cross country evidence. J Financ Econ Policy 10(3):369–385. https://doi.org/10.1108/JFEP-11-2016-0073
Google Scholar
-
Shi XY, Xu YZ (2023) Evaluating the equitable carbon reduction effect of Pilot “low-carbon City Policy——Based on the perspective of industrial carbon emissions. J Public Manag 20(1):84-96+173. https://doi.org/10.16149/j.cnki.23-1523.20221128.010
Google Scholar
-
Song JK, Zhang KX, Cao ZJ (2017) Provincial allocation of carbon emission quotas-under the fusion of fairness and efficiency. J Arid Land Resour Environ 31(5):7–13. https://doi.org/10.13448/j.cnki.jalre.2017.136
Google Scholar
-
Song M, Zhou P, Si HT (2021) Financial technology and enterprise total factor productivity——perspective of “enabling” and credit rationing. China Ind Econ 4:138–155. https://doi.org/10.19581/j.cnki.ciejournal.2021.04.006
Google Scholar
-
Sun H, Liu YY (2016) Analysis on inter-regional differences of carbon emissions and loss and profit deviation in China. Manag Rev 28(10):89–96. https://doi.org/10.14120/j.cnki.cn11-5057/f.2016.10.008
Google Scholar
-
Tang TW, Jiang XJ, Zhu KW et al. (2024) Effects of the promotion pressure of officials on green low-carbon transition: evidence from 277 cities in China. Energy Econ 129:107159. https://doi.org/10.1016/j.eneco.2023.107159
Google Scholar
-
Tu ZG, Wang K, Shen RJ (2022) Economic growth and pollution reduction: an integrated analytical framework. Econ Res J 57(8):154–171
Google Scholar
-
Wang J, Wang J, Li ZG (2022a) Development of digital finance and carbon emission of household consumption. Financ Econ 4:118–132. https://doi.org/10.3969/j.issn.1000-8306.2022.04.009
Google Scholar
-
Wang WJ, Kong XX (2022) Analysis on China’s provincial carbon quota allocation based on the 2030 carbon peak goal. J Quant Tech Econ 39(7):113–132. https://doi.org/10.13653/j.cnki.jqte.2022.07.002
Google Scholar
-
Wang WL, Wang JL, Wu HT (2024) The impact of energy-consuming rights trading on green total factor productivity in the context of digital economy: evidence from listed firms in China. Energy Econ 131:107342. https://doi.org/10.1016/j.eneco.2024.107342
Google Scholar
-
Wang XL, Fan G, Hu LP (2021) Marketization index of China’s provinces: Neri report 2021. Social Sciences Academic Press (China), Beijing
-
Wang YZ, Wang YF, Xu H et al. (2022b) Ambient air pollution and socioeconomic status in China. Environ Health Perspect 130(6):67001. https://doi.org/10.1289/EHP9872
Google Scholar
-
Wu HT, Li YW, Hao Y et al. (2020) Environmental decentralization, local government competition, and regional green development: evidence from China. Sci Total Environ 708:135085. https://doi.org/10.1016/j.scitotenv.2019.135085
Google Scholar
-
Wu YL, Huang SL (2022) The effects of digital finance and financial constraint on financial performance: firm-level evidence from China’s new energy enterprises. Energy Econ 112:106158. https://doi.org/10.1016/j.eneco.2022.106158
Google Scholar
-
Zhang JJ, Yu LC, Bi Q, Pan J (2016) Media supervision, environmental regulation and firm green investment. J Shanghai Univ Financ Econ 18(5):13. https://doi.org/10.16538/j.cnki.jsufe.2016.05.008
Google Scholar
-
Zhang XJ (2023) Do a good job of digital finance article to speed up the construction of a strong financial country. Guangming Daily:11
-
Zhang Y, Wang JJ, Cheng Y (2022) Spatiotemporal characteristics of China’s industrial carbon emission performance and influence mechanism of technological innovation. Resour Sci 44(7):1435–1448. https://doi.org/10.18402/resci.2022.07.10
Google Scholar
-
Zhang YJ, Liu Z, Zhang H, Tan TD (2014) The impact of economic growth, industrial structure and urbanization on carbon emission intensity in China. Nat Hazards 73(2):579–595. https://doi.org/10.1007/s11069-014-1091-x
Google Scholar
-
Zhao H, Yang YR, Li N et al. (2021) How does digital finance affect carbon emissions? Evidence from an emerging market. Sustainability 13(21):12303. https://doi.org/10.3390/su132112303
Google Scholar
-
Zhou R, Wang C, Xu T, Wang D (2014) A summary for the international conference of low carbon development and green economy. Econ Res J 49(11):184–188
Google Scholar
-
Zhu ZJ, Yuan YM, Xu M, Chai BF (2024) Digital finance, path breakthrough and high-quality innovation in manufacturing. J Quant Technol Econ 4:68–88. https://doi.org/10.13653/j.cnki.jqte.20240226.007
Google Scholar
Author information
Authors and Affiliations
Contributions
Heyu Lu: Conceptualization, methodology, data collection, software, visualization, writing—original draft preparation, supervision, writing—reviewing and editing. Zongfa Wu: Methodology, theoretical and supervision.
Corresponding author
Ethics declarations
Competing interests
The authors declare no competing interests.
Ethical approval
This article does not contain any studies with human participants performed by any of the authors.
Informed consent
This article does not contain any studies with human participants performed by any of the authors.
Additional information
Publisher’s note Springer Nature remains neutral with regard to jurisdictional claims in published maps and institutional affiliations.
Rights and permissions
Open Access This article is licensed under a Creative Commons Attribution-NonCommercial-NoDerivatives 4.0 International License, which permits any non-commercial use, sharing, distribution and reproduction in any medium or format, as long as you give appropriate credit to the original author(s) and the source, provide a link to the Creative Commons licence, and indicate if you modified the licensed material. You do not have permission under this licence to share adapted material derived from this article or parts of it. The images or other third party material in this article are included in the article’s Creative Commons licence, unless indicated otherwise in a credit line to the material. If material is not included in the article’s Creative Commons licence and your intended use is not permitted by statutory regulation or exceeds the permitted use, you will need to obtain permission directly from the copyright holder. To view a copy of this licence, visit http://creativecommons.org/licenses/by-nc-nd/4.0/.
Reprints and permissions
About this article
Cite this article
Lu, H., Wu, Z. Digital finance and equitable industrial carbon emissions: an empirical analysis of Chinese cities.
Humanit Soc Sci Commun 12, 175 (2025). https://doi.org/10.1057/s41599-025-04508-5
-
Received: 14 June 2024
-
Accepted: 28 January 2025
-
Published: 09 February 2025
-
DOI: https://doi.org/10.1057/s41599-025-04508-5
发表回复