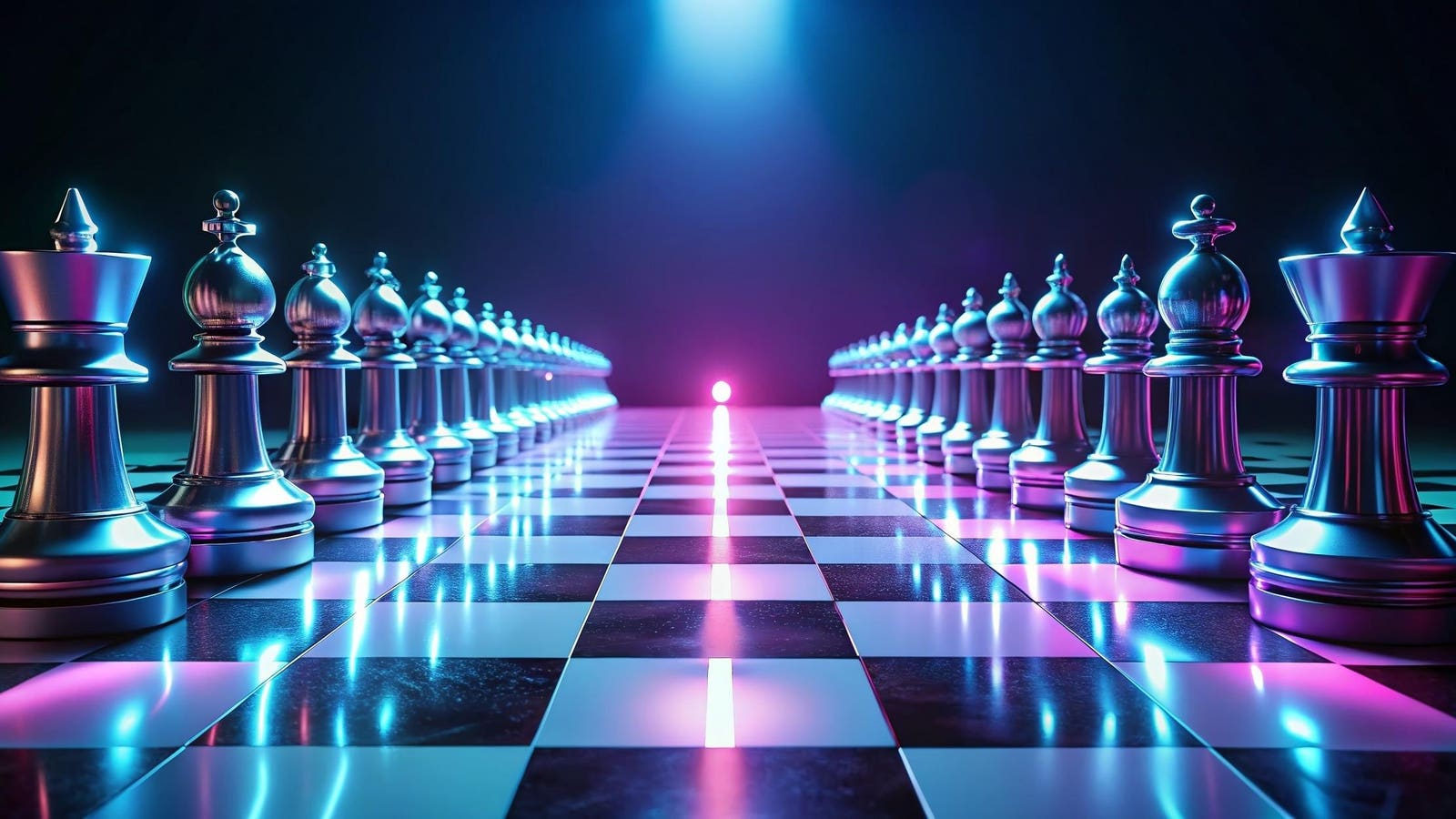
Video games have played an important role in the development of AI. Many early demonstrations of machine learning involved teaching computers to play games. Eventually, Google Deepmind’s mastery of the game Starcraft 2 was taken as proof that machines could now compete with us across many fields in which we were previously undisputed champions.
Now, games are being used as a testbed for exploring some of the most exciting new areas in AI, including autonomous agents, real-world robots and perhaps even the quest for AGI.
At this year’s Game Developer’s Conference, Google’s DeepMind AI division demonstrated its research into what it calls Scalable Instructable Multiworld Agents (SIMA).
The idea is to show that machines can navigate and learn inside the 3D worlds of video game environments. They can then use what they’ve learned to navigate entirely different worlds and tasks, all with their own rules, using whatever tools are available to them to solve problems.
It might sound like child’s play, but this research could dramatically impact the development of the agentic AI we’ll use in our work and personal lives. So let’s take a look at what it could mean, and whether it could even solve the ultimate AI challenge of creating machines capable of adapting to any situation, much like humans can.
Virtual Worlds
Video games provide a great environment for training AI because the variety of tasks and challenges is almost infinite. Importantly, the player usually solves these challenges using a standard set of tools, all accessed via the game controller.
This corresponds well with the way AI agents tackle problems by choosing which tools to use from a pre-defined selection.
Game worlds also provide safe, observable and scalable environments where the effects of subtle changes to variables or behavior can be explored at little real-world cost.
DeepMind’s SIMAs were trained across nine different video game environments, taken from popular games including No Man’s Sky, Valheim and Goat Simulator. The agents were given the ability to interact and control the games using natural language commands like “pick up the key” or “move to the blue building.”
Among the standout findings, the research showed that the agents are highly effective at transferable learning—taking what they learn in one game and using it to get better at another.
This was backed up by observations that agents trained to play eight of the nine games performed better at the one game they were untrained on than specialized agents solely trained on the one game.
This dynamic learning ability will be critical in a world where agents are working alongside us, helping us explore, interpret and understand messy real-world problems and situations.
But what about looking a little further ahead, to a time when it’s commonplace for robots to help us out with physical tasks as well as digital ones?
Physical Robots
The development of real-world robots that carry out physical tasks has accelerated in the last decade, hand-in-hand with the evolution of AI. However, they are still generally only used by large businesses due to the high cost of training them for specialist roles.
Using virtual and video game environments could dramatically lower this cost. The theory is that transferable learning will enable physical robots to use their hands, arms or whatever tools they have to tackle many physical challenges, even if they haven’t come across them before.
For example, a robot that effectively learns how to use its hands to work in a warehouse might also learn how to use them to build a house.
Before it released ChatGPT, OpenAI demonstrated research in this field. Dactyl is a robotic hand, trained in virtual simulated environments, that learned how to solve a Rubik’s Cube. This was one of the first demonstrations of the potential of transferring skills learned in virtual environments to complex physical-world tasks.
More recently, Nvidia has developed its Isaac platform expressly for the purpose of training robots to “learn to learn” how to carry out real-world tasks inside virtual environments.
Today, physical AI-assisted robots are put to work in warehouse roles, agriculture, healthcare, deliveries, and many other jobs. In most cases, however, these robots are still doing tasks they were specifically trained for—at enormous expense by companies with very deep pockets.
But new models of “affordable” robots are on the horizon. Tesla plans to manufacture thousands of its Optimus robots this year and assign many of them to work in its factories. And Chinese robotics developer Unitree recently unveiled a $16,000 humanoid robot that can turn its hand to many tasks.
With the price of robots falling and their AI brains becoming more powerful by the day, walking, talking humanoid robots could be stepping out of science fiction into everyday reality sooner than we think.
Toward AGI?
Almost 30 years ago, machines scored their first big win over humans by defeating Gary Kasparov at Chess. Few would have predicted then that a computer would exist that could beat world champions not just at one game, but at any game.
This ability to “generalize” information by taking knowledge from one task and using it to solve an entirely different one is traditionally exclusive to humans, but that could be changing.
All of this will be hugely interesting to those chasing the holy grail of AI development, artificial general intelligence (AGI).
Evidence that agents like DeepMind’s SIMAs are able to transfer learning from one virtual game environment to another suggests they may be developing some of the qualities needed for AGI. It demonstrates that they are progressively building competencies that can be applied to solving future problems.
Google, along with OpenAI, Anthropic and Microsoft, have all stated that developing AGI is their eventual goal, and it’s clearly the logical endpoint of the current focus on agentic intelligence. With video games, could another part of the puzzle be in place?
发表回复